Table of content:
Population & Sample in Statistics: Definition, Difference & Example
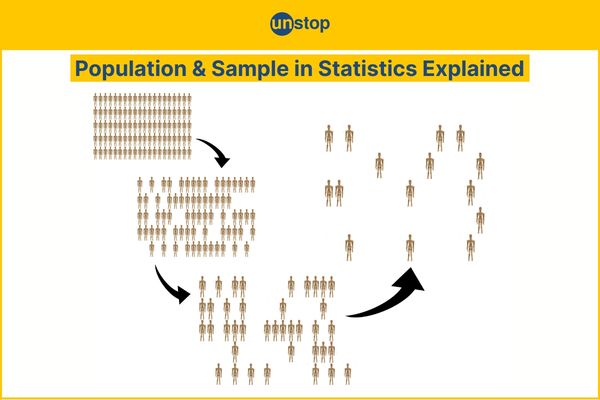
In statistics, the terms "population" and "sample" are fundamental concepts used to describe the scope and scale of data collection and analysis. These terms help us distinguish between the entirety of a group being studied and a smaller subset chosen from that group.
Understanding the difference between a population and a sample and knowing when to use each is crucial for conducting meaningful research and drawing reliable conclusions.
What is a Population in Statistics?
In statistics, a population encompasses all the individuals, items, or events that share a common characteristic or are of interest in a specific study. It is the complete set from which researchers aim to gather insights.
Populations can vary in size, ranging from small groups, such as the employees of a particular company, to vast entities, such as the global human population.
Population Examples in Statistics
- All the students enrolled in a university.
- Every household in a city.
- All smartphones are manufactured by a specific company in a year.
Studying an entire population can provide accurate and comprehensive data, but this approach is often impractical due to constraints such as time, cost, and logistics. However, in certain situations, such as a small, well-defined group (e.g., committee members), studying the entire population may be feasible.
What is a Sample in Statistics?
In statistics, a sample is a smaller, manageable subset of a population selected for analysis. Samples are used when it is impossible or impractical to study the entire population.
Researchers analyze the sample and use the results to make inferences about the broader population.
Sample Examples in Statistics
- A survey of 500 students from a university with 20,000 students.
- A random selection of 1,000 households from a city with 1 million households.
- A quality check on 200 smartphones out of 10,000 produced.
The primary goal of sampling is to ensure that the subset accurately represents the population. This is achieved by employing proper sampling techniques and avoiding bias wherever possible.
Key Differences Between Population Vs. Sample
Feature |
Population |
Sample |
Definition |
Entire group under study |
Subset of the population |
Size |
Larger, encompassing all members |
Smaller, a manageable number |
Data Collection |
Often uses census or complete enumeration |
Relies on sampling methods |
Accuracy |
Provides precise, comprehensive insights |
Results are estimates, subject to error |
Feasibility |
Expensive, time-consuming |
Cost-effective and practical |
Examples |
All students in a university |
500 students surveyed from the university |
Importance of Representativeness in Sample & Population
For a sample to provide reliable insights into the population, it must be representative. A representative sample mirrors the population's characteristics, ensuring that the findings are generalizable. Bias in sampling can lead to skewed results, which might mislead decision-making processes.
Example of Representativeness
Imagine a company that wants to gauge employee satisfaction. If it surveys only employees from one department, the results may not represent the views of the entire workforce. To avoid bias, it should include employees from various departments, job levels, and locations.
Visualizing representativeness using a diagram that shows the population and a well-chosen sample can further clarify this concept for readers.
Common Sampling Techniques, Applications & Challenges
Sampling Technique
- Simple Random Sampling: Every member of the population has an equal chance of being selected. For example, drawing names from a hat.
- Stratified Sampling involves dividing the population into subgroups (strata) based on shared characteristics and taking samples from each stratum. For instance, students are sampled based on their academic year.
- Systematic Sampling: Members are selected at regular intervals from an ordered list. An example is choosing every 10th name from a list of customers.
- Cluster Sampling: The population is divided into clusters, and a random sample of clusters is studied. For example, selecting a few neighborhoods to survey in a city.
- Convenience Sampling: Samples are taken from a group that is readily available, such as surveying passersby at a mall. However, this method risks bias and is less reliable.
Sampling Applications
- Healthcare: Researchers studying the effects of a new drug often test a sample of patients before generalizing the results to the larger population. This ensures safety and efficacy.
- Marketing: Companies survey a sample of customers to understand their preferences and improve their products. One example is A/B testing of website layouts.
- Government Policy: National censuses often rely on sampling methods to supplement complete enumeration efforts, saving resources while maintaining accuracy.
Challenges in Sampling
While sampling is practical, it is not without challenges. These include:
- Sampling Bias: Occurs when the sample does not represent the population accurately, such as over-representing one demographic group.
- Non-Response: When selected individuals do not participate, the results can potentially be skewed. Follow-up efforts can mitigate this.
- Sample Size: A small sample may fail to capture the population’s diversity, while an overly large sample may be resource-intensive. Determining an optimal sample size using statistical formulas is crucial.
Conclusion
The distinction between population and sample is a cornerstone of statistical analysis. While studying an entire population offers comprehensive insights, it is often impractical, making sampling an essential tool. By employing appropriate sampling methods, avoiding bias, and ensuring representativeness, researchers can draw meaningful conclusions about populations. These concepts are vital in fields ranging from healthcare to marketing, where data-driven decisions impact outcomes.
Understanding these principles is not just academic but critical for anyone working with data. Whether you are a student, researcher, or professional, mastering the balance between population and sample can enhance your ability to make informed and effective decisions.
Frequently Asked Questions (FAQs)
1. What is the difference between a population and a sample in statistics?
A population includes all members of a specified group being studied, while a sample is a smaller subset selected from the population for analysis. Samples are used to make inferences about the entire population when studying the whole group is impractical.
2. Why is sampling necessary in research?
Sampling is necessary because studying an entire population is often resource-intensive, time-consuming, and sometimes impossible. A well-chosen sample allows researchers to gather insights efficiently and cost-effectively while ensuring results that can be generalized to the population.
3. What are the characteristics of a good sample?
A good sample should be representative of the population, unbiased, and of sufficient size to capture the population's diversity. It should be selected using appropriate sampling techniques to ensure accuracy and reliability.
4. What are some common sampling techniques?
Common sampling techniques include:
- Simple Random Sampling: Equal chances for all members to be selected.
- Stratified Sampling: Division of the population into subgroups, with samples taken from each.
- Systematic Sampling: Selection at regular intervals.
- Cluster Sampling: Studying randomly selected clusters from the population.
- Convenience Sampling: Using easily accessible subjects (less reliable).
5. How does sample size affect the reliability of research?
Sample size directly impacts the reliability of research findings. A sample that is too small may fail to represent the population, leading to inaccurate conclusions. Conversely, an excessively large sample may waste resources without significantly improving accuracy. Determining the optimal sample size involves balancing precision with practicality.
Suggested reads:
- Deep Web: Unveiling The Hidden Layers Of The Internet
- What Is A CISCO Packet Tracer? Features, Applications, Benefits
- File Sharing In Operating Systems For Collaboration And Efficiency
- Quantum Networking: Bridging The Future Of Communication
- What Is NVMe? Why Every Gamer, Data Scientist, and Tech Enthusiast Needs It