The future of Data Scientists: Will the next decade witness a complete extinction?
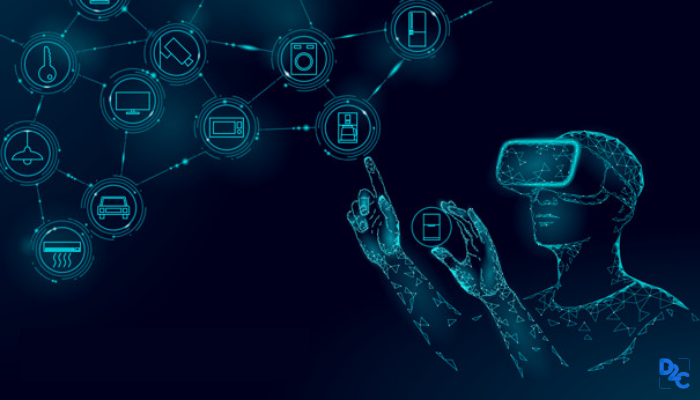
Data has become an indispensable part of the 21st Century with our society witnessing rapid digitalization in the last couple of years. Even basic surveys that were earlier carried out in paper format are now done using digital forms.
In today's majorly AI-driven world, regardless of whether you work as a recruiting HR, sales marketing expert-or in the manufacturing, agriculture, or advertising sector-data is playing a huge role in defining and transforming your work sector.
The easy accessibility of abundance of data implies that there's a decent possibility that your work capacity would benefit from outside intervention with present-day apparatuses like Data Science and Machine Learning.
But is the mere availability of data and knowing how to implement ML models will take your company to greater heights via the route of automation?
Undoubtedly Artificial Intelligence is expanding its domain day by day, which has resulted in the easy availability of base-level data science to almost everyone in society.
What does it mean?
- Earlier people weren't consuming so much data online. As time progressed and the internet craze got hold of almost everyone, one of the basic prerequisites to step into the field of Data Sciences, i.e. the availability of data is no longer a barrier.
- With a ceaseless stock of new data companies popping up, you can get access to any data without spending even having to spend a thousand bucks sometimes.
- Another traditional prerequisite was the need to have expensive and robust on-site hardware on board, which has been rendered practically non-existent with the introduction of all-powerful cloud computing.
At last, balancing the trinity of essentials is the ability and skill to carry out, which has ostensibly become the most omnipresent part of information science. Due to the democratized availability of huge data sets, basic know-how of data science, and related software on the internet, implementing any Machine Learning model has become a matter of merely a "few seconds" and a "few lines of codes".
Isn't easy access to information and know-how tutorials related to any domain a good thing?
As per Mikhail Mew, a Researcher, Investor, and Data Scientist, “in todays' highly impatient digitized society, people are majorly eyeing instant satisfaction and fulfillment of their agendas. Though ease of access isn't impending on face esteem, underneath the stunning cluster of programming libraries and sparkly new models, the genuine motivation behind Data Sciences has gotten clouded and, now and again, even neglected.”
One must not only run complex ML models without any sense of purpose, thought process, or direction attached to it. Implementing multiple models only for the sake of decorating your resume with our skill-sets or to improve a self-assertive exhibition metric will only belittle the importance of Data Sciences, which in reality should be used as an apparatus to tackle genuine issues.
Sadly, the current standard has gotten more centered around practicing and coding Data Sciences as opposed to applying it to solve genuine issues. In this way, the entire concept behind the advent of Data Science and Artificial Intelligence is disappearing.
Well, this misalignment is one of the symptoms of the decline of data scientists. Confused? Let's take a higher perspective to understand the same.
What does Data Science imply?
For starters, Data Science is the art of extracting relevant information, major insights, and knowledge from a given set of data. Hence, the end result of any data science project is mostly an array of slides or a PPT that basically concludes the entire scenario for business heads to make decisions, or for a group of technical and product experts to conclude how to work on a site.
The key steps directing the workflow of any data science project is mainly:
- Collecting a lot of data (for verified sources)
- Analyzing data and iterating it multiple times to develop a sound understanding of the data
- Suggesting hypotheses or actions that include periodically analyzing and updating data.
Data Science and Artificial Intelligence Industrial Revolution
When one says that he wants to become a Data scientist, the main question that arises is “data scientists of what?” Data Science has the inquisitive differentiation of being one amongst a handful of work fields that leaves the specialist without any specific industrial domain. Hence, the expansive utilization of Data Science and Information Technology ends up being a two-sided deal.
- On one side, an amazing tool stash can be applied to any industry where information is produced and caught. On the other, the overall pertinence of these devices implies that users are more inclined towards deploying this system without having any substantial knowledge about the field.
- When Data Science was beginning to ascend in the industry, bosses raced to bridle this beginning innovation without completely getting what it was and how it very well may be completely incorporated into their organization. Hence, having complete knowledge about a particular sector wasn't considered necessary as nothing was generalized back then.
- Nowadays, a tremendous amount of data is generated every passing minute in every sector and industry. Since the stretch of data is ubiquitous and boundless, it's quite obvious that a percentage of that data ought to be irrelevant from a profit generation point of view.
- Also, different companies have different domains of expertise and manufacturing, so to make fruitful utilization of the data related to that sector, it's necessary to have a piece of sound background knowledge about that industry and how one can efficiently employ a Machine-learning model to make the company scale new heights.
What are the newly set standards of a Data Scientist?
With the advent of time, the industry standards and the broad definition of a Data Scientist have witnessed a change.
- Organizations currently look towards setting up a wholesome Data Science framework by hiring large and consistent deep-seated teams, benchmarked by setting up industry guidelines.
- The squeezing recruiting process has moved to critical thinkers, analysts, and problem solvers who comprehend all the nuances of the business, its area of expertise along with its various collaborators.
- Hence, the mere know-how of several software packages or the ability to disgorge a couple of lines of code would not get the job done.
- A Data Scientist is no longer characterized by his coding skills, which is confirmed by the rising prominence of no code, auto ML arrangements like Datarobot, Rapid Miner, and Alteryx.
Today’s AI Era ≠ Any Industry + Deep Learning (or mere knowledge of Data Science software and coding abilities) |
It implies:
|
What can be concluded on the fate of Data Scientists?
Obviously, Data Science can never go extinct. However, the titular positions and roles of Data Science will surely notice a dynamic change. In another decade or so, data-savvy industry domain specialists, business analysts, and field experts will become Data Science experts by getting trained in AI and ML.
These specialists will be able to permeate analysis with their deep-rooted industrial knowledge, regardless of if they can code. Their titles will mirror their aptitude instead of the methods by which they execute it, be it recruiting HRs, CEOs, compliance specialists, or investment analysts.
For more such interesting stories, read:
- Leveraging technology in the supply chain process | Walmart
- Meet the national winners of AB InBev Maverick 2.0 Bot-a-thon
- Painting the success story of Team CODE CHARISMA in Myntra hacker-ramp WeForShe hackathon- By Anika Jagati from VIT, Chennai
- This 20 year old Indian girl gets awarded INR 22 lakhs by Microsoft for finding a bug
Login to continue reading
And access exclusive content, personalized recommendations, and career-boosting opportunities.
Comments
Add comment