Data Science Hackathon vs Normal Hackathon: Which Is The Better Choice?
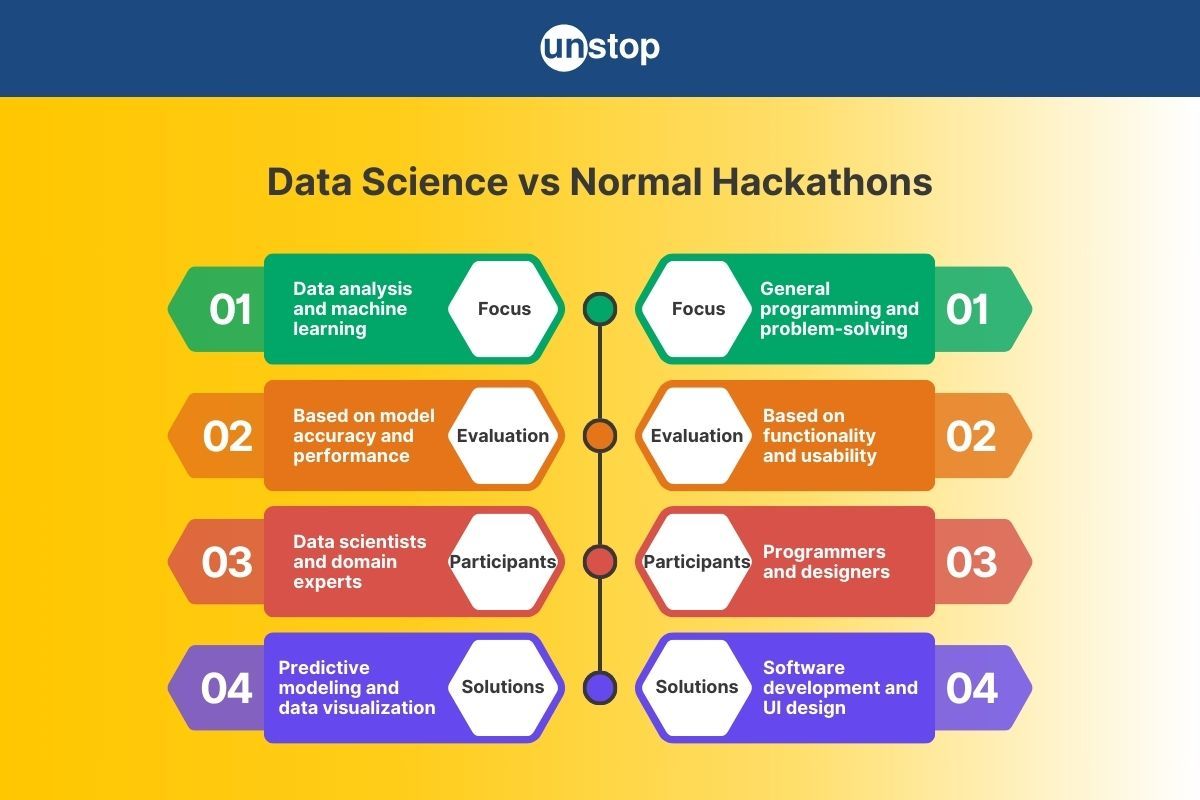
Data science hackathons are competitive events where participants use their data analysis and programming skills to solve complex problems. These hackathons provide a platform for data scientists, programmers, and analysts to showcase their abilities and collaborate with others. Unlike normal hackathons, data science hackathons often involve working with large datasets and applying machine learning algorithms to find innovative solutions.
Hosting data science hackathons can be highly beneficial for companies. These events provide a platform for companies to tap into the expertise of data scientists and discover innovative solutions to their business challenges. By hosting a data science hackathon, companies can gain insights into their data, uncover hidden patterns, and develop data-driven strategies. Additionally, these hackathons can serve as a recruitment tool, allowing companies to identify and connect with top talent in the field of data science.
Explore the Unstop platform for other effective hiring solutions, along with engagement and assessment tools.
Key Differences between Data Science Hackathons and Normal Hackathons
Here’s a snapshot of a data science hackathon vs normal hackathon:
Criteria | Data Science Hackathons | Normal Hackathons |
---|---|---|
Focus and Purpose | Focus on data analysis and machine learning and emphasis is on data cleaning and preprocessing | Focus on general programming and problem-solving and emphasis is on code optimization and efficiency |
Datasets | Participants work with large datasets | Participants work with smaller datasets or no datasets |
Evaluation Parameters | Evaluation based on model accuracy and performance | Evaluation based on functionality and usability |
Skill Requirements | Requires knowledge of statistics and machine learning algorithms | Requires knowledge of programming languages and algorithms |
Type of Participants | Often involves collaboration between data scientists and domain experts | May involve collaboration between programmers and designers |
Solutions provided | Solutions often involve predictive modeling and data visualization | Solutions often involve software development and user interface design |
Let’s understand the differences in detail:
Focus and Purpose
Data science hackathons primarily revolve around analyzing and interpreting data, while normal hackathons typically involve developing software or creating prototypes. In a data science hackathon, participants dig deep into datasets to extract insights and build predictive models. On the other hand, normal hackathons may focus on building functional applications or solving specific problems through coding.
Skill Requirements
Data science hackathons require a strong understanding of statistical analysis and machine learning techniques, which is not typically needed in normal hackathons. Participants in data science hackathons must possess knowledge of programming languages like Python or R, as well as expertise in data manipulation and visualization. In contrast, normal hackathon participants may need proficiency in coding languages relevant to their project but might not require advanced statistical analysis skills.
Evaluation Criteria
The outcomes of data science hackathons are often measured by the accuracy or effectiveness of the models created. The success of a data science project depends on how well it performs when applied to new data. In contrast, normal hackathon projects may be evaluated based on functionality, user experience, or the completion of specific tasks within a given time frame.
Collaboration vs Competition
While collaboration is essential in both types of hackathons, the nature of collaboration differs slightly. Data science hackathons tend to encourage teamwork among participants with diverse skill sets since different perspectives can lead to better insights and model development. Normal hackathons also promote collaboration; however, there may be more competition involved as teams strive to create unique solutions that stand out from others.
Data Science Hackathon vs Normal Hackathon: Which is Better for Companies?
When it comes to determining whether a data science hackathon or a normal hackathon is better for companies, it ultimately depends on the specific goals and needs of the organization. Both types of hackathons have their advantages and can bring value to companies in different ways.
A data science hackathon is particularly beneficial for companies that heavily rely on data analysis and want to leverage the power of data science to gain insights and make data-driven decisions. Data science hackathons provide a platform for teams to tackle complex data problems, develop innovative algorithms, and create predictive models.
They can help companies uncover hidden patterns in data, optimize processes, and gain a competitive edge in their respective industries.
A normal hackathon, which is not limited to data science, allows participants to work on a wide range of projects and ideas. This type of hackathon can be beneficial for companies that are looking for innovative solutions to various challenges, not necessarily limited to data-related problems. Normal hackathons provide a creative and collaborative environment where participants can brainstorm ideas, develop prototypes, and showcase their skills.
They can help companies foster innovation, discover new talent, and generate fresh ideas that can be applied to different areas of their business.
Choosing Data Science Hackathon vs Normal Hackathon: Factors to Consider
When choosing between a data science hackathon and a normal hackathon, several factors should be considered:
-
Goals and Objectives: Companies should clearly define their goals and objectives for hosting a hackathon. If the primary objective is to solve data-related challenges and leverage data science techniques, a data science hackathon would be more suitable. However, if the goal is to explore a broader range of ideas and solutions, a normal hackathon might be a better choice.
-
Skill Sets: Consider the skill sets of the participants and the expertise required for the specific challenges. Data science hackathons typically require strong analytical and programming skills, while normal hackathons may involve a broader range of skills such as design, marketing, and business development.
-
Resources and Infrastructure: Evaluate the resources and infrastructure available to support the hackathon. Data science hackathons often require access to large datasets, specialized tools, and computational resources. Normal hackathons, on the other hand, may require more general resources such as collaboration spaces and prototyping materials.
-
Collaboration and Networking Potential: Consider the level of collaboration and networking opportunities provided by each type of hackathon. Data science hackathons often involve interdisciplinary teams working together to solve complex problems, which can foster collaboration and networking among participants with diverse backgrounds. Normal hackathons may also provide networking opportunities, but the focus may be more on individual or small team projects.
-
Impact and Innovation: Assess the potential impact and level of innovation that can be achieved through each type of hackathon. Data science hackathons have the potential to generate innovative solutions to data-related challenges and drive data-driven decision-making within companies. Normal hackathons may lead to creative and innovative ideas, but the impact may be more varied depending on the nature of the challenges.
-
Time and Cost: Consider the time and cost implications of hosting the hackathons. Data science hackathons often require more time for data exploration, analysis, and modeling, as well as the development and testing of algorithms. The complexity of data-related challenges can also increase the time required for solution development. Additionally, the need for specialized tools and computational resources may incur additional costs. On the other hand, normal hackathons may have a shorter time frame and lower costs as they often focus on rapid prototyping and idea generation. The time and cost considerations should align with the company's resources and priorities when deciding between a data science hackathon and a normal hackathon.
In conclusion, the key differences between data science hackathons and normal hackathons lie in the specific focus on data analysis, machine learning, and problem-solving techniques that are required in the former. Overall, data science hackathons offer a unique opportunity for individuals to enhance their skills and knowledge in the field of data science and for firms to find the right talent, establish themselves as industry leaders, and build a talent pipeline.
Frequently Asked Questions (FAQs)
Q1: How do I choose the right platform for hosting a data science hackathon?
Choosing the right platform for hosting a data science hackathon involves considering factors such as available datasets, tools, community support, and ease of use. Look for platforms that provide diverse datasets relevant to your problem statement along with pre-built libraries or frameworks that can streamline your workflow.
Looking for the right platform to conduct a data science hackathon? Explore Unstop’s Data Science Assessment Platform.
Q2: What’s the one key difference between a data science hackathon and a normal hackathon?
The key difference between a data science hackathon and a normal hackathon is the focus on data analysis and machine learning techniques in a data science hackathon.
Q3: Why is collaboration important in data science hackathon teams?
Collaboration is important in data science hackathon teams because it allows individuals to leverage their diverse skills and perspectives. By working together, team members can combine their expertise to develop more robust and creative solutions.
Q4: Are data science hackathons suitable for beginners?
Yes, data science hackathons can be beneficial for beginners as they provide a hands-on learning experience. Participants can learn from more experienced team members, gain exposure to real-world problems, and build their confidence in applying data science techniques.
Q5: How can data science hackathons help in hiring?
Data science hackathons can be a valuable tool for recruiters in the hiring process. Firstly, hackathons provide recruiters with an opportunity to directly observe candidates' skills and problem-solving abilities in a real-world setting. By participating in a hackathon, candidates can showcase their technical skills, teamwork, and creativity, which are essential qualities for a data science role. Additionally, hackathons allow recruiters to assess candidates' ability to work under pressure and meet tight deadlines, which are crucial skills in a fast-paced data science environment. Furthermore, hackathons provide recruiters with a chance to identify top talent and potential future hires. By observing how candidates perform in a competitive setting, recruiters can identify individuals who stand out and demonstrate exceptional skills and potential. Overall, data science hackathons offer recruiters a unique and effective way to evaluate candidates' abilities and make informed hiring decisions.
Suggested Reads:
Login to continue reading
And access exclusive content, personalized recommendations, and career-boosting opportunities.
Blogs you need to hog!
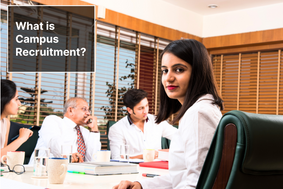
What is Campus Recruitment? How To Tap The Untapped Talent?
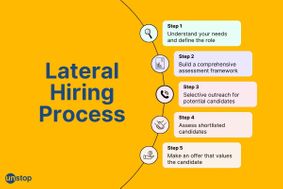
Lateral Hiring: A Complete Guide To The Process, Its Benefits, Challenges & Best Practices
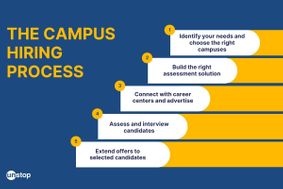
Step-By-Step Approach To Building A Winning Campus Recruitment Strategy
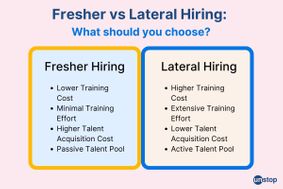
Comments
Add comment