Table of content:
- What Is Mean Deviation?
- How to Calculate Mean Deviation for Ungrouped Data
- How to Calculate Mean Deviation for Grouped Data
- Mean Deviation for Discrete & Continuous Frequency Distribution
- Mean Deviation Using Different Measures
- Pros of Mean Deviation
- Cons of Mean Deviation
- Select Practice Questions with Answers
- Conclusion
- Frequently Asked Questions (FAQs)
Mean Deviation: Definition, Formula, Solved Examples & Practice Questions (With Answers)
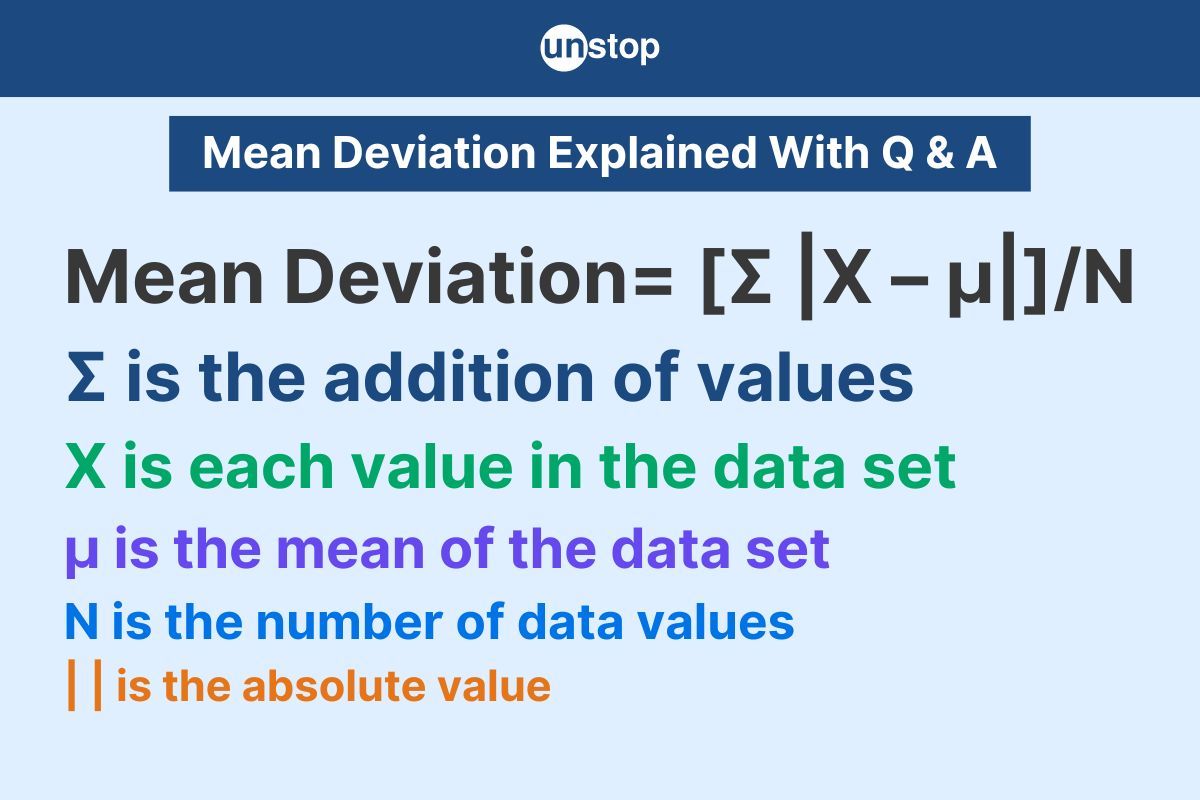
In Mathematics, deviation shows how much a value differs from what's expected. Calculating mean deviation provides insights into the consistency or variability within a dataset, offering valuable information for decision-making processes and trend analysis.
Let's learn more about its definition, formula, and examples.
What Is Mean Deviation?
To begin with, let us understand the definition of mean deviation:
Mean Deviation Definition
Mean deviation, also known as the average deviation, is a measure of dispersion that calculates the average difference between each data point and the mean. It is calculated by finding the average of the absolute differences between each value and the mean.
Formula Of Mean Deviation
The formula for the mean deviation (also known as the average absolute deviation) from the mean is:
Mean Deviation = [Σ |X – µ|]/N
- Σ is the addition of values
- X is each value in the data set
- µ is the mean of the data set
- N is the number of data values
- | | is the absolute value, which ignores the “-” symbol
How to Calculate Mean Deviation for Ungrouped Data
To find the mean deviation of ungrouped data, start by calculating the average of all the numbers in the set. Next, determine the difference between each number and the mean to get the deviations.
Next, find the absolute value of each deviation. Add these absolute deviations together and divide by the total number of observations to obtain the mean deviation.
Application Example
For instance, consider a dataset: 10, 15, 20, 25. The mean is (10+15+20+25)/4 = 17.5. Deviations are (-7.5), (-2.5), 2.5, and 7.5, respectively.
Absolute deviations are 7.5, 2.5, 2.5, and 7.5. The sum of these absolute deviations is 20 divided by 4 (total observations) equals a mean deviation of 5.
How to Calculate Mean Deviation for Grouped Data
In grouped data, frequencies represent the number of observations falling within each interval. These frequencies play a crucial role in determining the mean deviation for grouped datasets. By multiplying each frequency with its corresponding class mid-point, we can calculate the deviation from the mean.
Application Example
To illustrate the calculation of mean deviation for grouped data, consider a dataset with intervals and frequencies. Start by finding the midpoint of each class interval. Multiply these midpoints by their respective frequencies. Next, find the sum of these products. Divide this sum by the total frequency to obtain the mean deviation.
Mean Deviation for Discrete & Continuous Frequency Distribution
Discrete Frequency Distribution
Discrete data can be counted and has distinct values (e.g., number of students, cars). Discrete data is always ungrouped.
Example: The number of siblings a person has is discrete and ungrouped data.
Calculation:
- Calculate the arithmetic mean (X̄) of the data.
- Find the deviation of each value (x) from the mean: |x - X̄|.
- Multiply each deviation by its corresponding frequency (f).
- Sum up the products obtained in step 3.
- Divide the sum by the total number of observations (N).
Formula: MD = Σf|x - X̄| / N
Example: Calculating Mean Deviation
Discrete Data: Let's consider the number of children in 5 families: 2, 3, 1, 4, 2.
- Calculate the mean: (2+3+1+4+2)/5 = 2.4
- Calculate deviations from the mean: |2-2.4|, |3-2.4|, |1-2.4|, |4-2.4|, |2-2.4|
- Calculate the mean deviation: (0.4+0.6+1.4+1.6+0.4)/5 = 0.8
Mean deviation = 0.8
Continuous Frequency Distribution
Continuous data is measurable and can take any value within a range. For example, height, weight, time. Continuous data can be either grouped or ungrouped.
Example: The heights of students in a class can be presented as ungrouped (individual heights) or grouped into intervals (e.g., 150-160 cm, 160-170 cm).
Calculation:
- Find the mid-point (m) of each class interval.
- Calculate the arithmetic mean (X̄) using the mid-points and frequencies.
- Find the deviation of each mid-point from the mean: |m - X̄|.
- Multiply each deviation by its corresponding frequency (f).
- Sum up the products obtained in step 4.
- Divide the sum by the total number of observations (N).
Formula: MD = Σf|m - X̄| / N
Key point to remember: The formula for calculating mean deviation remains the same for both discrete and continuous data, with the only difference being the use of mid-points for continuous data.
Mean Deviation Using Different Measures
Let us also look at how mean deviation is calculated using different measures:
Mean vs. Median vs. Mode
When calculating mean deviation about the mean, the steps involve finding the absolute values of data values from the mean, summing these values, and dividing by the total number of data points.
On the other hand, when calculating mean deviation about the median, the process requires finding the absolute differences between each data value and the median.
In contrast, determining mean deviation based on the mode involves computing the absolute values of data values from the mode. The formula then consists of summing these absolute distances and dividing by the total number of data points.
To sum it up:
- Mean deviation about Mean (MD about X̄) = Σ|x - X̄|/n
- Mean deviation about Median (MD about M) = Σ|x - M|/n
- Mean deviation about Mode (MD about Z) = Σ|x - Z|/n
- Where:
- x = individual data point
- X̄ = arithmetic mean
- M = median
- Z = mode
- n = number of data points
- Σ = summation
Key Points
- Absolute values: Deviations are always positive as negative signs are ignored.
- Central tendency: It measures dispersion around a central value.
- Less sensitive to outliers: Compared to standard deviation, it is less affected by extreme values.
- Less common: It's not as widely used as standard deviation in statistical analysis.
Pros of Mean Deviation
Let us study some of the advantages of mean deviation:
Intuitive Interpretation
Mean deviation, also known as average deviation, offers a straightforward way to understand the spread of data. It calculates the average distance between each data point and the mean. This simplicity makes it easier for individuals without statistical expertise to grasp the variability in a dataset.
Robustness To Outliers
One significant advantage of using mean deviation is its resilience to outliers. Mean deviation considers all data points equally. This property makes it a more reliable measure of variability when dealing with datasets that contain outliers or skewed distributions.
Ease Of Computation
Calculating mean deviation involves simpler arithmetic. By summing the absolute differences between each data point and the mean, dividing by the total number of observations yields the mean deviation.
Cons of Mean Deviation
Let us also look at some of the limitations of mean deviation:
Lack Of Sensitivity
Mean deviation has limitations in accurately representing the variability of a dataset. One key drawback is its lack of sensitivity to extreme values. It can be heavily influenced by these extreme values, leading to a skewed representation.
Inability To Account For Variability
Another limitation of mean deviation is its inability to account for the squared differences from the mean, treating them as absolute values. This characteristic makes mean deviation less effective in capturing the full range of variability within a dataset.
Click here to learn more about various topics related to quantitative aptitude, including mean deviation.
Select Practice Questions with Answers
Question 1: What is the mean deviation of the data set {7, 3, 13, 5, 23, 20, 39, 23, 40, 23, 14, 12, 56, 23, 29}?
a) 10.67
b) 12.76
c) 10
d) 13.67
Answer: a) 10.67
Explanation: Mean x=23.3; Find the absolute difference between each data point and the mean. Mean deviation=162/15. Therefore, the mean deviation≈10.67
Question 2: If the mean deviation of the data set {4, 8, 6, 12, 5, 4, 5, 4} is 2, what is the mean of the data set?
a) 5
b) 6
c) 7
d) 8
Answer: a) 5
Explanation:
We know that Mean Deviation (MD) = Σ|x - Mean|/n
Where:
- Σ = Summation
- x = individual data point
- Mean = Average of the data set
- n = number of data points
We are given MD = 1.75 and the data set. We need to find the mean.
Let the mean be 'x'.
So, 1.75 = (|4-x| + |8-x| + |6-x| + |12-x| + |5-x| + |4-x| + |5-x| + |4-x|) / 8
=> 14 = |4-x| + |8-x| + |6-x| + |12-x| + |5-x| + |4-x| + |5-x| + |4-x|
By inspection, we can see that x = 5 satisfies the equation.
Therefore, the mean of the data set is 5.
Question 3: What is the mean deviation of the data set {10, 9, 8, 7, 6, 5, 4, 3, 2, 1}?
a) 2.5
b) 3.5
c) 4.5
d) 5.5
Answer: a) 2.5
Explanation: The mean deviation is the average of the absolute deviations from the mean, so we first find the mean (5.5) and then find the average of the absolute deviations.
Question 4: If the mean deviation of the data set (7, 9, 2, 4, 4, 4, 5, 5) is 1.5, what is the mean of the data set?
a) 4
b) 5
c) 6
d) 7
Answer: b) 5
Explanation: The mean deviation is the average of the absolute deviations from the mean, so we can work backwards to find the mean.
Question 5: What is the mean deviation of the given data set (2, 2, 2, 2, 2)?
a) 0
b) 1
c) 2
d) 3
Answer: a) 0
Explanation: The mean deviation is the average of the absolute deviations from the mean, so we first find the mean (2) and then find the average of the absolute deviations.
Question 6: If the mean deviation of the given data set (1, 1, 2, 2, 3, 3, 4, 4) is 1, what is the mean of the data set?
a) 2
b) 2.5
c) 3
d) 3.5
Answer: b) 2.5
Explanation: The mean deviation is the average of the absolute deviations from the mean, so we can work backwards to find the mean.
Question 7: A data set has a mean of 30 and a mean deviation of 5. If we add a new data point which is 50, what can be said about the new mean deviation?
a) It will decrease
b) It will increase or stay the same
c) It will stay the same
d) It will become zero
Answer: b) It will increase or stay the same
Explanation: Adding a data point that is further from the mean than the current mean deviation will increase the mean deviation or keep it the same.
Question 8: A data set has a mean of 40 and a mean deviation of 10. If we multiply each data point by 3, what will be the new mean deviation?
a) 50
b) 20
c) 40
d) 30
Answer: d) 30
Explanation: The mean deviation is affected by multiplying or dividing all data points by a constant. It will also be multiplied by the same constant.
Question 9: If the mean deviation of a data set is 10 and the mean of the data set is 20, what will be the new mean deviation if each data point is increased by 5?
a) 10
b) 20
c) 15
d) 25
Answer: a) 10
Explanation: The mean deviation is not affected by adding or subtracting a constant from all data points.
Question 10: The mean deviation of a data set is 4. If all the data points are multiplied by 3, what will be the new mean deviation?
a) 20
b) 18
c) 16
d) 12
Answer: d) 12
Explanation: The mean deviation is affected by multiplying or dividing all data points by a constant. It will also be multiplied by the same constant.
Conclusion
Mean deviation is a useful tool for analyzing how spread out data points are. Calculating mean deviation for grouped and ungrouped data can provide important insights into the distribution of the data. Despite its limitations, mean deviation offers a simple yet effective way to measure dispersion, providing a different angle to interpret data beyond the traditional measures.
Keep practising calculations and analyzing different datasets to master the application of mean deviation effectively.
Frequently Asked Questions (FAQs)
1. What is the mean deviation?
Mean deviation, a measure of dispersion, shows the average variance between individual data points and the dataset mean. It gives an idea of how widely the values deviate from the central point.
2. How is mean deviation calculated for ungrouped data?
For ungrouped data, calculate mean deviation by finding the absolute differences between each data point and the mean, then averaging these differences. The formula involves summing up |x - mean| for all data points and dividing by the total number of observations.
3. Can mean deviation be calculated for grouped data?
Mean deviation can also be determined for grouped data by adjusting the formula to consider the frequency of each class interval. This modified formula accounts for the average absolute deviations from the group's mean.
4. What are some advantages of using mean deviation?
Mean deviation is simple to grasp and compute, making it handy for swiftly evaluating the diversity within a set of data. It assigns the same importance to all data points and is less influenced by extreme values when compared to standard deviation.
5. Are there limitations to using mean deviation?
One limitation of mean deviation is that it doesn't consider whether differences from the mean are positive or negative, potentially cancelling out deviations. It also lacks certain mathematical properties that make standard deviation preferable in some statistical analyses.
Suggested reads:
- Profit And Loss- Basic Concepts, Formulas, Questions & Answers
- Difference Between Simple Interest And Compound Interest Formula
- Time, Speed And Distance- Formula With Aptitude Question & Answer
- Train Problems: Concept & Selected Practice Question With Answer
- Squares And Cubes: Roots, Formula, Calculation, Question & Answer
Instinctively, I fall for nature, music, humour, reading, writing, listening, travelling, observing, learning, unlearning, friendship, exercise, etc., all these from the cradle to the grave- that's ME! It's my irrefutable belief in the uniqueness of all. I'll vehemently defend your right to be your best while I expect the same from you!
Login to continue reading
And access exclusive content, personalized recommendations, and career-boosting opportunities.

Subscribe
to our newsletter
Comments
Add comment